Optimization of a cogenerating HRSG
· Key To Energy ·
HelioSwitch has collaborated with Key To Energy to develop an algorithm for economic optimization of the operations of gas turbines with heat recovery steam generation and cogeneration.
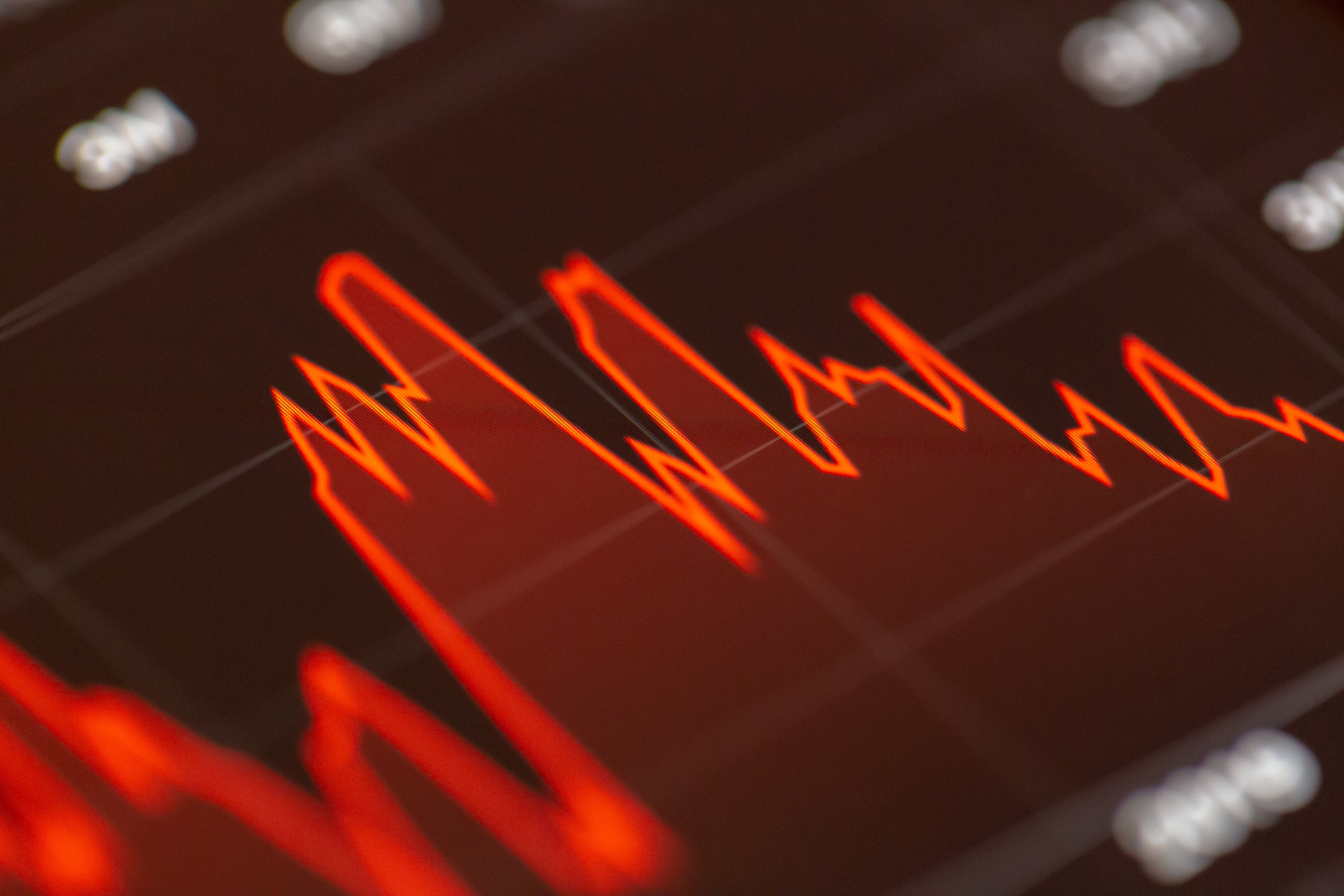
In this project we were tasked to design, develop, test, and ship an economic optimization algorithm for a cogenerating power plant.
The challenge involved modeling the power plant's thermodynamics, along with its physical and operational constraints, in order to define a suitable action space for optimization.
Our team's unique mix of skills in both machine learning and the physical and engineering aspects of the system were instrumental in tackling this complex problem and developing an effective solution.